스타트업 인턴 일기 [01화]에서 이어집니다...
https://jhklee-coder.tistory.com/91
지난 스타트업 인턴 일기 [01화]에 이어 이번 [02화]는 'LangGraph', '지식그래프'의 주요 개념에 대해 다루고자 한다. 주된 내용으로는 LangGraph와 Neo4j, 지식그래프(Knowledge Graph)를 결합하여 end-to-end 로 이어지는 LangGraph + Neo4j 의 구현이며, 전체 코드 예제도 추가했다.
1️⃣ RAG
RAG(Retrieval-Augmented Generation)는 검색 증강 생성이라고 하는 대규모 언어 모델의 활용 기법 중 하나로, Fine-Tuning 과 더불어 최근 가장 많이 각광을 받고 있는 분야 중 하나다. LLM의 경우, 사전에 학습을 위해 엄청난 양의 데이터를 학습한다. 그 과정에서 100만 개, 500만 개, 많게는 10억, 70억 개의 매개변수가 가중치를 조절하면서 외부의 데이터를 모델에 적합한 형태로 학습하게 된다. Fine-Tuning은 이렇게 사전에 학습된 모델을 외부의 데이터를 갖고서 추가로 학습을 시키는 형태로, 일부 가중치를 조정하여 특정 영역(도메인)이나 기능에 더 적합하게 만든다.
쉽게 말해, 국, 영, 수, 과탐 전과목을 다 잘하던 학생이 대학에 가 물리학을 전공하는 것과 유사하다. 즉 모델이 특정 영역에 더 적합하고 더 똑똑하게 만드는 과정이 바로 fine-tuning(파인튜닝)이다. 파인튜닝의 장점은 특정 영역에서 더 높은 정확성과 유용함을 보인다는 점이다. 하지만 모델의 추가 학습을 위해 많은 시간과 비용을 요구하며, 새로운 데이터를 학습하는 과정에서 일반화 지식을 잃고, 복잡성만 증가한다던지, 여러 문제가 발생한다.
이를 보완하고, 또 기존에 LLM이 갖고 있던 근본적인 한계인 Hallucination을 극복하기 위해 등장한 방식이 바로 RAG다. RAG는 기존 언어 모델에 '검색(Retrieval)'의 기능을 결합해 사용자의 입력과 관련된 정보를 축적된 DB 등에서 검색한 뒤, 이를 기반으로 답변을 하는 구조다. 참고할 만한 자료를 검색해 그와 관련성이 높은 대답을 제공하는 방법이다. 이는 모델이 실시간 정보 검색을 통해 더 정확한 답변을 생성할 수 있게 하며, fine-tuning의 단점을 효율적으로 개선할 수 있는 장점을 제공한다.
✅ GraphRAG
실제 다양한 기업에서 RAG를 적용한 LLM 프레임워크를 활용해 자사의 AI 시스템을 구축하고, 서비스를 제공하고 있다. 일반적인 RAG 시스템의 경우 문서나 기타 형태의 데이터를 벡터 임베딩 형태로 변환한 뒤, 이를 vectorDB에 저장하여 필요할 때마다 유사성 검색을 수행해 LLM에 컨택스트를 주입하는 형태를 띄고 있다.
하지만 실제 비즈니스 환경에는 문서와 같은 비정형 텍스트 외에도 숫자(가격 정보 등), 집계, 관계와 같은 구조화된 속성 요소들이 많이 포함된다. 즉, 실제 데이터 시나리오를 거치면서 처리할 때 내부 chain 구조에서 쿼리의 정확성과 효율성이 저해되는 문제가 발생하게 된다.
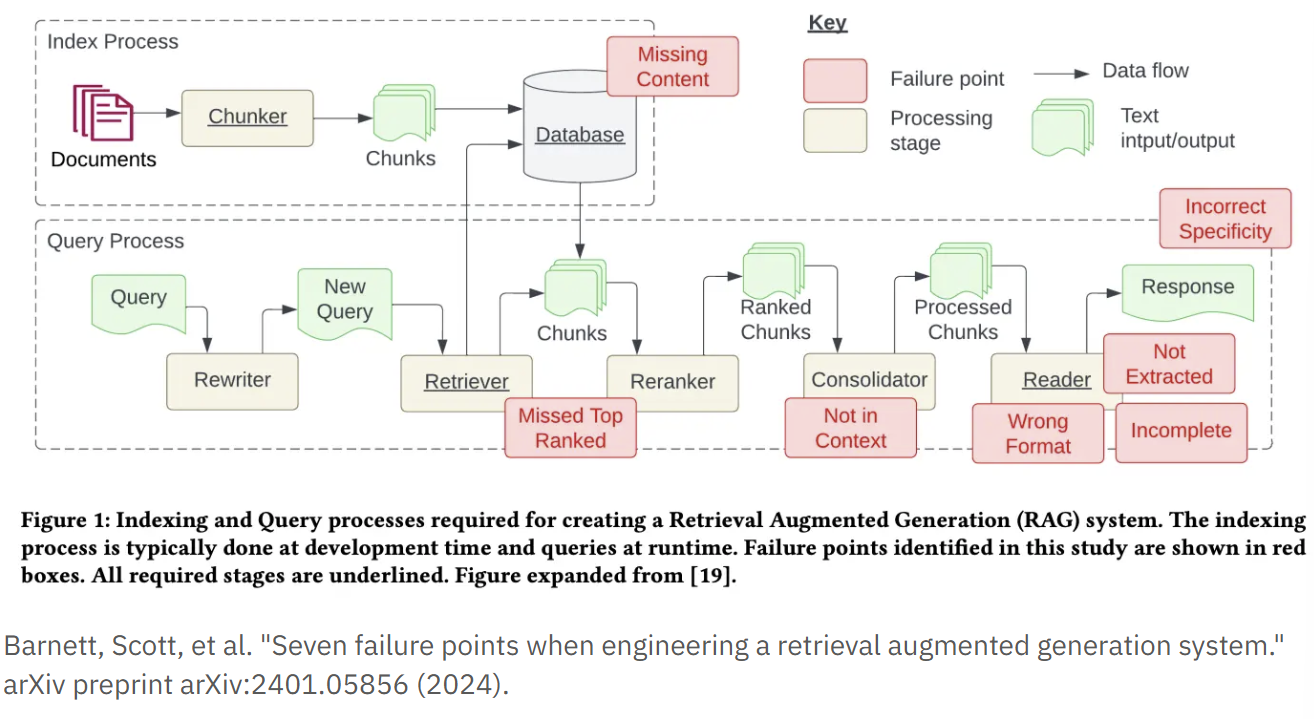
이외에도 retrieval 과정에서 vector DB에 저장된참고 자료를 제대로 가져오지 못한다던지, 사용자 입력에 대한 불완전한 응답을 제공한다던지 등 여러 한계가 존재한다. 이와 같은 기존 벡터 기반의 RAG 한계점을 보완하기 위해 지식 그래프 개념 기반의 GraphRAG가 등장했다.
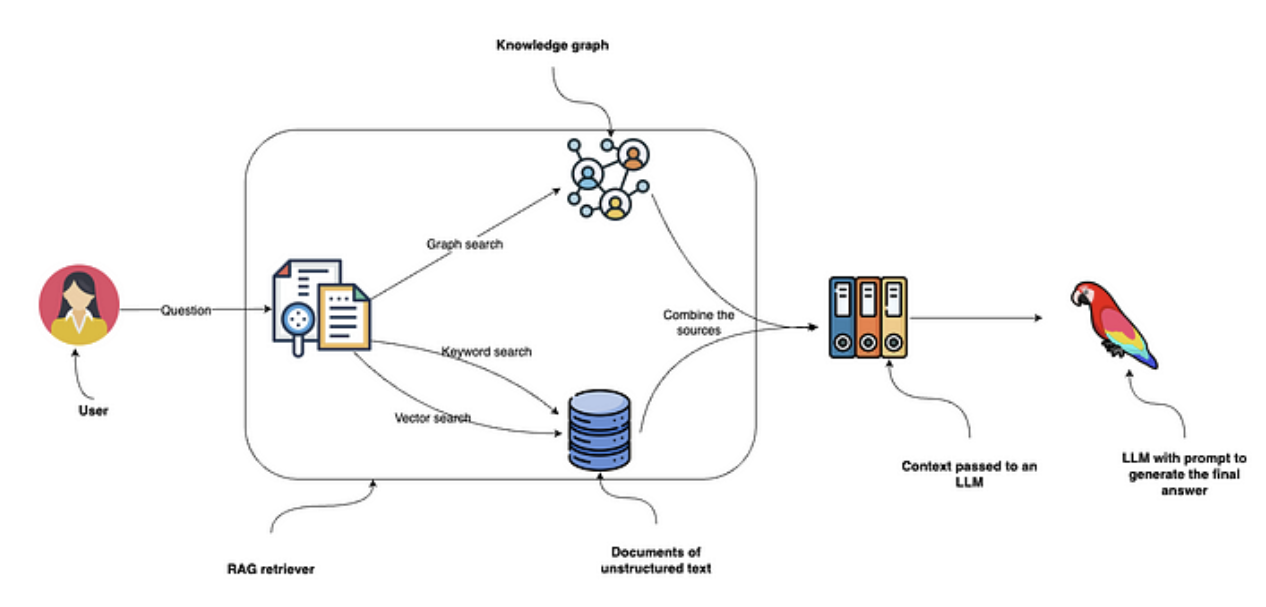
✅ Neo4j
지식 그래프(Knowledge Graph)를 구축해 지식을 검색하는 형태의 GraphRAG는 구조화된 데이터 엔티티(entity) 간 관계를 관리하고 활용하기 용이하다. 특히 그래프 형태로 상호 연결된 정보(노드, 엣지 등)들을
표현하고 저장하는데 지식 그래프의 데이터 구조와 GraphRAG 방식은 굉장히 효율적으로 동작할 수 있다. 그리고 이번엔 이 그래프 구조를 잘 표현하고 저장할 수 있는 Neo4j와의 결합을 해보고자 한다.
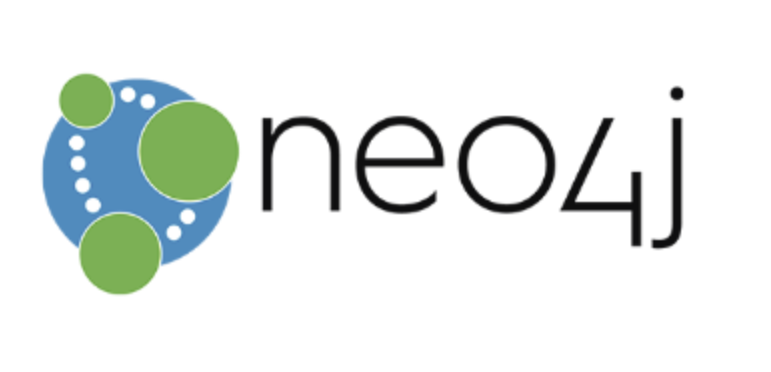
특징
1. 대규모 그래프 분석 및 데이터 시각화를 지원하는 확장성이 뛰어난 그래프 데이터베이스
2. Cypher 쿼리 언어, 내장된 시각화 도구 및 커뮤니티 지원
2️⃣ LangGraph
지난 포스트 중, langchain 에 대해 간단한 소개를 한 적이 있다. https://jhklee-coder.tistory.com/88
LangChain (랭체인)
랭체인(LangChain)은 대규모 언어 모델과 앱의 통합을 간소화하는 SDK*다. 최근 LLM 모델의 부상과 함께 그 활용성의 측면에서 랭체인의 범용성 또한 퍼지고 있다. 새로운 오픈소스 모델의 출시나 상
jhklee-coder.tistory.com
최근 기존의 LLM 통합과 체인을 구성하는 LangChain 기본 기능을 넘어 더 복잡한 내부 워크플로우와 정교한 의사결정 프로세스를 요구하는 경우가 많아지고 있다. 기존의 간단한 체인 구조와 애플리케이션을 구성하는데 중점을 두었던 Langchain의 한계를 좀 더 보완하고, 더 복잡한 프레임워크를 제공하는 것이 바로 LangGraph다. (이번 인턴 활동에서 가장 많이 활용해야 하는 영역이 바로 graph이기 때문에, LangGraph 라이브러리의 활용은 필수가 될 듯하다...)
LangGraph vs LangChain
주요 목적 | 복잡한 워크플로우 및 의사결정 프로세스 구현 | LLM 통합 및 체인 구성 |
구조 | 그래프 기반 | 체인 및 에이전트 기반 |
상태 관리 | 명시적이고 세밀한 제어 | 암시적이고 자동화된 관리 |
유연성 | 높음 (커스텀 로직 쉽게 구현) | 중간 (미리 정의된 컴포넌트 중심) |
학습 곡선 | 상대적으로 가파름 | 상대적으로 완만함 |
용도 | 복잡한 AI 시스템, 다중 에이전트 | 간단한 LLM 애플리케이션, RAG |
출처 - https://wikidocs.net/261585
✅ LangGraph의 주요 특징
1. 그래프 구조의 WorkFlow
2. 명시적인 상태 관리 (State Management)
3. 다중 에이전트 시스템 (Multi-agent)
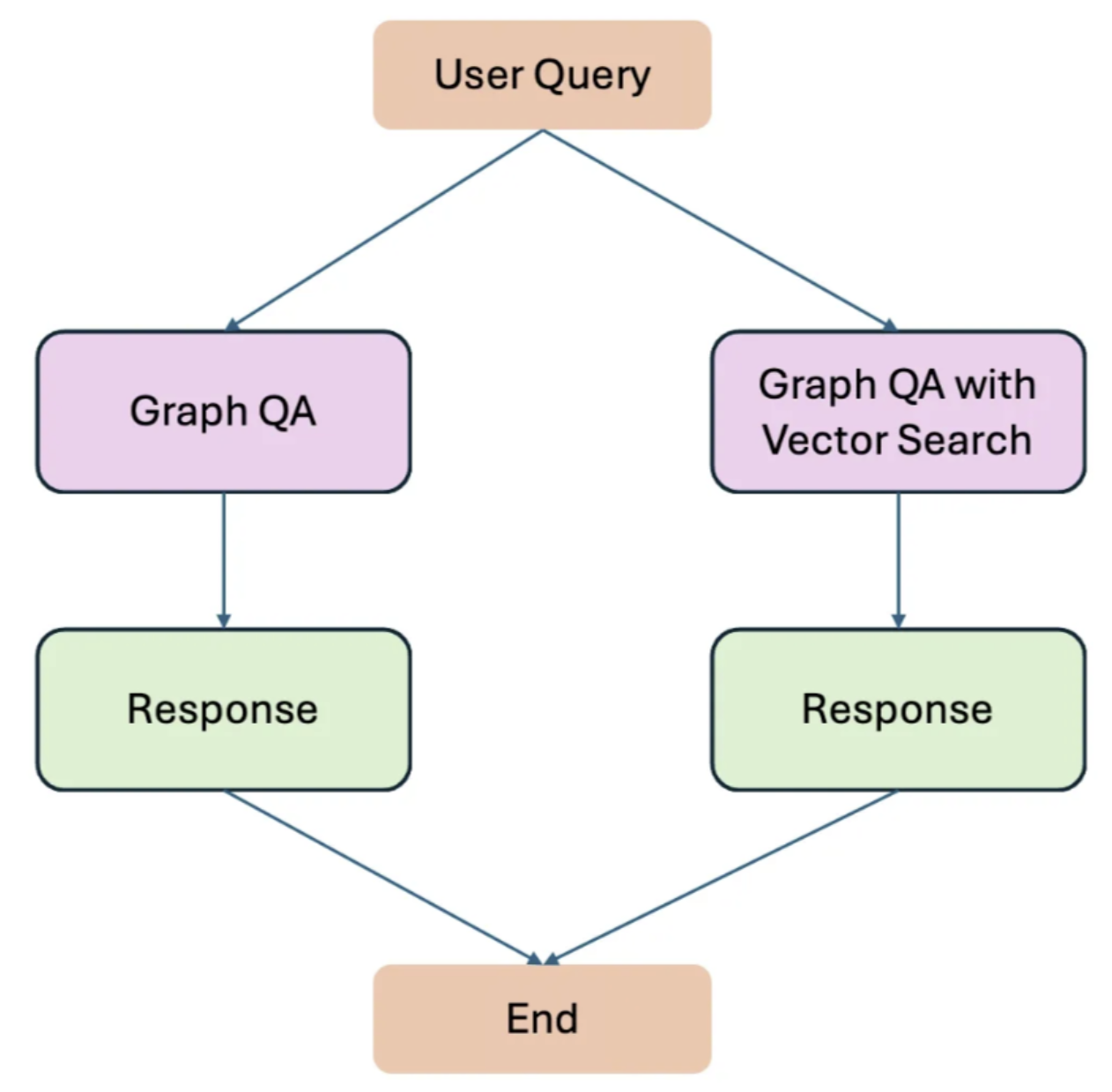
3️⃣ GraphRAG + LangGraph + Neo4j
✅ Neo4j 인스턴스 생성 및 Data Ingestion
1. langchain 및 neo4j 라이브러리 설치
# 라이브러리 및 패키지 설치
!pip install langchain
!pip install langchain_openai
!pip install neo4j
!pip install pyvis
2. Neo4j 인스턴스 생성
# Neo4j Sandbox Web browser DB 사용 예정
from langchain_community.graphs import Neo4jGraph
graph = Neo4jGraph(url = "bolt://...", username = "neo4j", password = "...", refresh_schema = False)
3. data를 그래프 구조로 ingest 하기 위한 함수 정의
import csv
import numpy as np
from neo4j import GraphDatabase
# Node : Retailer, Product, Supplier, Distributor, Manufacturer
NODES_CSV = "C:/LangGraph_Tutorial/.../nodes.csv"
# Edge : supplies, manufactures, offers
RELATIONSHIPS_CSV = "C:/LangGraph_Tutorial/.../relationships.csv"
# 데이터를 ingestion 하기 위한 함수
def get_label_for_type(node_type):
mapping = {
"Supplier": "Supplier", # 공급자
"Manufacturer" : "Manufacturer", # 생산자
"Distributor" : "Distributor", # 유통업자
"Retailer" : "Retailer", # 소매업자
"Product" : "Product" # 제품
}
return mapping.get(node_type, "Entity")
# 노드 정보 적재
def ingest_nodes(driver):
with driver.session() as session:
with open(NODES_CSV, mode = 'r', encoding = "utf-8") as f:
reader = csv.DictReader(f)
for row in reader:
node_id = row['id:ID']
name = row['name']
node_type = row['type']
location = row['location']
supply_capacity = np.random.randint(1000, 50001)
description = row['description']
label = get_label_for_type(node_type)
if location.strip():
# cypher 쿼리
query = f"""
MERGE (n:{label} {{id:$id}})
SET n.name = $name, n.location = $location,
n.description = $description, n.supply_capacity = $supply_capacity
"""
params = {
"id": node_id,
"name" : name,
"location" : location,
"description" : description,
"supply_capacity" : supply_capacity
}
else :
query = f"""
MERGE (n:{label} {{id:$id}})
SET n.name = $name
"""
params = {"id": node_id, "name": name}
session.run(query, params)
# 엣지(관계) 정보 적재
def ingest_relationships(driver):
with driver.session() as session:
with open(RELATIONSHIPS_CSV, mode='r', encoding='utf-8') as f:
reader = csv.DictReader(f)
for row in reader:
start_id = row[':START_ID']
end_id = row[':END_ID']
rel_type = row[':TYPE']
product = row['product']
if product.strip():
# cypher 쿼리
query = f"""
MATCH (start {{id:$start_id}})
MATCH (end {{id:$end_id}})
MERGE (start)-[r:{rel_type} {{product:$product}}]->(end)
"""
params = {
"start_id": start_id,
"end_id": end_id,
"product": product
}
else:
query = f"""
MATCH (start {{id:$start_id}})
MATCH (end {{id:$end_id}})
MERGE (start)-[r:{rel_type}]->(end)
"""
params = {
"start_id": start_id,
"end_id": end_id
}
session.run(query, params)
def create_indexes(driver):
with driver.session() as session:
for label in ["Supplier", "Manufacturer", "Distributor", "Retailer", "Product"]:
session.run(f"CREATE CONSTRAINT IF NOT EXISTS FOR (n:{label}) REQUIRE n.id IS UNIQUE")
4. Neo4j Sandbox web browser DB 연결 후 ingestion 수행
driver = GraphDatabase.driver(uri = "bolt://...", auth = ("neo4j", "..."))
create_indexes(driver)
ingest_nodes(driver)
ingest_relationships(driver)
print("Data ingestion complete!")
driver.close()
# => Data ingestion complete!

✅ Langchain 프레임워크 결합
1. Vector Embeddings 결합
import os
os.environ["OPENAI_API_KEY"] = "sk-..." # openai api key 입력
2. 구조화된 데이터 검색과 구조화되지 않은 텍스트 검색을 결합
from langchain_openai import OpenAIEmbeddings
from langchain_community.vectorstores import Neo4jVector
# neo4j 에 embedding 된 schema 구조.
embedding = OpenAIEmbeddings(model="text-embedding-3-small")
neo4j_vector = Neo4jVector.from_existing_graph(
url = "bolt://...",
username = "neo4j",
password = "...",
embedding=embedding,
index_name="supply_chain",
node_label="Supplier", # embedding 저장소를 supplier 노드에 제한
text_node_properties=["description"],
embedding_node_property="embedding",
)
=> Neo4j에서 semantic similarity search를 통해 그래프 형태의 구조화된 데이터를 검색 + 구조화되지 않은 텍스트 데이터(벡터)의 검색 기능을 결합.
✅ 구조화된 Query 처리를 위한 Tooling 작업
1. 숫자 필터링, 공급업체 계산, 목록 작성 등을 위한 도구 정의
# 입력 스키마 정의
from pydantic import BaseModel, Field
from typing import Optional, Dict, List
# 공급업체의 카운팅을 위한 class이자 Tool 정의
class SupplierCountInput(BaseModel):
min_supply_amount: Optional[int] = Field(
description="Minimum supply amount of the suppliers" # Supplier의 최소 공급량
)
max_supply_amount: Optional[int] = Field(
description="Maximum supply amount of the suppliers" # Supplier의 최대 공급량
)
grouping_key: Optional[str] = Field(
description="The key to group by the aggregation", # 최종 집계를 위한 키
enum=["supply_capacity", "location"]
)
2. 공급업체 계산을 위한 함수 정의
import re
from langchain_core.tools import tool
def extract_param_name(filter: str) -> str:
pattern = r'\$\w+'
match = re.search(pattern, filter) # filter 문자열에서 패턴을 검색
if match:
return match.group()[1:]
return None
@tool("supplier-count", args_schema=SupplierCountInput) # -> 도구 이름을 supplier-count tool로 등록
def supplier_count(
min_supply_amount: Optional[int], # 최소 공급량 필터
max_supply_amount: Optional[int], # 최대 공급량 필터
grouping_key: Optional[str],
) -> List[Dict]: # => return 값 : 공급업체 집계 리스트
"""Calculate the count of Suppliers based on particular filters"""
# -> 필터 조건 정의
filters = [
("t.supply_capacity >= $min_supply_amount", min_supply_amount), # 최소 공급량 조건
("t.supply_capacity <= $max_supply_amount", max_supply_amount) # 최대 공급량 조건
]
# condition에 포함된 변수명, 값을 매핑
params = {
extract_param_name(condition): value
for condition, value in filters if value is not None
}
# None이 아닌 조건들을 AND로 연결, Where 절 생성
where_clause = " AND ".join(
[condition for condition, value in filters if value is not None]
)
# 기본 cypher 쿼리 생성
cypher_statement = "MATCH (t:Supplier) " # "Supplier" 노드 매칭
if where_clause:
cypher_statement += f"WHERE {where_clause} "
return_clause = (
f"t.{grouping_key}, count(t) AS supplier_count"
if grouping_key
else "count(t) AS supplier_count"
)
cypher_statement += f"RETURN {return_clause}"
print(cypher_statement) # Debugging output
return graph.query(cypher_statement, params=params)
1) extract_param_name 함수
- 필터 조건에서 매개변수 이름을 추출.
- $min_supply_amount와 같은 조건에서 변수 이름을 가져옴.
2) supplier_count 함수
- 주어진 필터를 기반으로 공급업체를 세는 Cypher 쿼리를 생성.
- 위치와 같은 속성으로 결과를 그룹화(선택 사항).
3. 공급업체 목록 도구 정의
class SupplierListInput(BaseModel):
sort_by: str = Field(
description="How to sort Suppliers by supply capacity", enum=['supply_capacity']
)
k: Optional[int] = Field(
description="Number of Suppliers to return"
)
description: Optional[str] = Field(
description="Description of the Suppliers"
)
min_supply_amount: Optional[int] = Field(
description="Minimum supply amount of the suppliers"
)
max_supply_amount: Optional[int] = Field(
description="Maximum supply amount of the suppliers"
)
4. 공급업체 데이터를 검색하고 정렬하는 도구 정의
@tool("supplier-list", args_schema=SupplierListInput) # supplier-list 라는 tool로 등록.
def supplier_list(
sort_by: str = "supply_capacity", # 공급 능력에 따라 정렬
k : int = 4,
description: Optional[str] = None,
min_supply_amount: Optional[int] = None,
max_supply_amount: Optional[int] = None,
) -> List[Dict]:
"""List suppliers based on particular filters"""
# Handle vector-only search when no prefiltering is applied
# -> description이 주어지고, 다른 필터링 조건이 없을 시 벡터 유사성 검색을 수행.
if description and not min_supply_amount and not max_supply_amount:
return neo4j_vector.similarity_search(description, k=k)
filters = [
("t.supply_capacity >= $min_supply_amount", min_supply_amount),
("t.supply_capacity <= $max_supply_amount", max_supply_amount)
]
params = {
key.split("$")[1]: value for key, value in filters if value is not None
}
where_clause = " AND ".join([condition for condition, value in filters if value is not None])
cypher_statement = "MATCH (t:Supplier) "
if where_clause:
cypher_statement += f"WHERE {where_clause} "
# Sorting and returning
cypher_statement += " RETURN t.name AS name, t.location AS location, t.description as description, t.supply_capacity AS supply_capacity ORDER BY "
if description:
cypher_statement += (
"vector.similarity.cosine(t.embedding, $embedding) DESC " # 코사인 유사도를 기반으로 정렬
)
params["embedding"] = embedding.embed_query(description)
elif sort_by == "supply_capacity":
cypher_statement += "t.supply_capacity DESC "
else:
# Fallback or other possible sorting
cypher_statement += "t.year DESC "
cypher_statement += " LIMIT toInteger($limit)"
params["limit"] = k or 4
print(cypher_statement) # Debugging output
data = graph.query(cypher_statement, params=params)
return data
- 공급업체 나열
- 공급 능력별 정렬
- 능력별 필터링
- description 제공 시 벡터 검색을 수행하는 도구
✅ LangChain & LangGraph 과의 결합
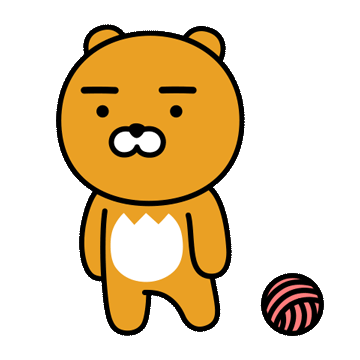
이번 주는 RAG 에 대한 주요 개념과 Graph를 활용한 RAG 기법의 핵심 기능 확장, 나아가 LangGraph를 활용한 파이프라인 구현을 해보았다. 아직 업무에 대한 이해와 LinkBrain 프로젝트의 방향성을 스스로 확립하지 못했다는 생각이 든다. 아직은 열심히 프레임워크와 툴, 핵심 로직 구현을 위한 공부에 열중할 때..!!!!
인턴 일기는 다음 글에 이어집니다...
3️⃣ Reference
https://medium.com/globant/langgraph-ai-agents-with-neo4j-knowledge-graph-7e688888f547
LangGraph AI Agents with Neo4j Knowledge Graph
A RAG System Built for Both Semantic Search and Structured Data Queries
medium.com
https://python.langchain.com/docs/tutorials/graph/
Build a Question Answering application over a Graph Database | 🦜️🔗 LangChain
In this guide we'll go over the basic ways to create a Q&A chain over a graph database. These systems will allow us to ask a question about the data in a graph database and get back a natural language answer. First, we will show a simple out-of-the-box opt
python.langchain.com
https://langchain-ai.github.io/langgraph/
Home
🦜🕸️LangGraph ⚡ Building language agents as graphs ⚡ Overview LangGraph is a library for building stateful, multi-actor applications with LLMs, used to create agent and multi-agent workflows. Check out an introductory tutorial here. LangGraph is
langchain-ai.github.io
https://github.com/sgautam666/my_graph_blogs/tree/main/neo4j_rag_with_langGraph
my_graph_blogs/neo4j_rag_with_langGraph at main · sgautam666/my_graph_blogs
A collection project that uses graph technology. Contribute to sgautam666/my_graph_blogs development by creating an account on GitHub.
github.com
'Experience' 카테고리의 다른 글
스타트업 인턴 일기 [04화] (0) | 2025.02.14 |
---|---|
스타트업 인턴 일기 [03화] (1) | 2025.02.06 |
스타트업 인턴 일기 [01화] (0) | 2025.01.15 |
경희대학교 데이터 분석 & AI 동아리 KHUDA 4th [교내활동] (1) | 2023.12.26 |
OUTTA 부트캠프(최종 프로젝트) / 후기 및 활동 내역 [대외활동] (0) | 2023.12.23 |
스타트업 인턴 일기 [01화]에서 이어집니다...
https://jhklee-coder.tistory.com/91
지난 스타트업 인턴 일기 [01화]에 이어 이번 [02화]는 'LangGraph', '지식그래프'의 주요 개념에 대해 다루고자 한다. 주된 내용으로는 LangGraph와 Neo4j, 지식그래프(Knowledge Graph)를 결합하여 end-to-end 로 이어지는 LangGraph + Neo4j 의 구현이며, 전체 코드 예제도 추가했다.
1️⃣ RAG
RAG(Retrieval-Augmented Generation)는 검색 증강 생성이라고 하는 대규모 언어 모델의 활용 기법 중 하나로, Fine-Tuning 과 더불어 최근 가장 많이 각광을 받고 있는 분야 중 하나다. LLM의 경우, 사전에 학습을 위해 엄청난 양의 데이터를 학습한다. 그 과정에서 100만 개, 500만 개, 많게는 10억, 70억 개의 매개변수가 가중치를 조절하면서 외부의 데이터를 모델에 적합한 형태로 학습하게 된다. Fine-Tuning은 이렇게 사전에 학습된 모델을 외부의 데이터를 갖고서 추가로 학습을 시키는 형태로, 일부 가중치를 조정하여 특정 영역(도메인)이나 기능에 더 적합하게 만든다.
쉽게 말해, 국, 영, 수, 과탐 전과목을 다 잘하던 학생이 대학에 가 물리학을 전공하는 것과 유사하다. 즉 모델이 특정 영역에 더 적합하고 더 똑똑하게 만드는 과정이 바로 fine-tuning(파인튜닝)이다. 파인튜닝의 장점은 특정 영역에서 더 높은 정확성과 유용함을 보인다는 점이다. 하지만 모델의 추가 학습을 위해 많은 시간과 비용을 요구하며, 새로운 데이터를 학습하는 과정에서 일반화 지식을 잃고, 복잡성만 증가한다던지, 여러 문제가 발생한다.
이를 보완하고, 또 기존에 LLM이 갖고 있던 근본적인 한계인 Hallucination을 극복하기 위해 등장한 방식이 바로 RAG다. RAG는 기존 언어 모델에 '검색(Retrieval)'의 기능을 결합해 사용자의 입력과 관련된 정보를 축적된 DB 등에서 검색한 뒤, 이를 기반으로 답변을 하는 구조다. 참고할 만한 자료를 검색해 그와 관련성이 높은 대답을 제공하는 방법이다. 이는 모델이 실시간 정보 검색을 통해 더 정확한 답변을 생성할 수 있게 하며, fine-tuning의 단점을 효율적으로 개선할 수 있는 장점을 제공한다.
✅ GraphRAG
실제 다양한 기업에서 RAG를 적용한 LLM 프레임워크를 활용해 자사의 AI 시스템을 구축하고, 서비스를 제공하고 있다. 일반적인 RAG 시스템의 경우 문서나 기타 형태의 데이터를 벡터 임베딩 형태로 변환한 뒤, 이를 vectorDB에 저장하여 필요할 때마다 유사성 검색을 수행해 LLM에 컨택스트를 주입하는 형태를 띄고 있다.
하지만 실제 비즈니스 환경에는 문서와 같은 비정형 텍스트 외에도 숫자(가격 정보 등), 집계, 관계와 같은 구조화된 속성 요소들이 많이 포함된다. 즉, 실제 데이터 시나리오를 거치면서 처리할 때 내부 chain 구조에서 쿼리의 정확성과 효율성이 저해되는 문제가 발생하게 된다.
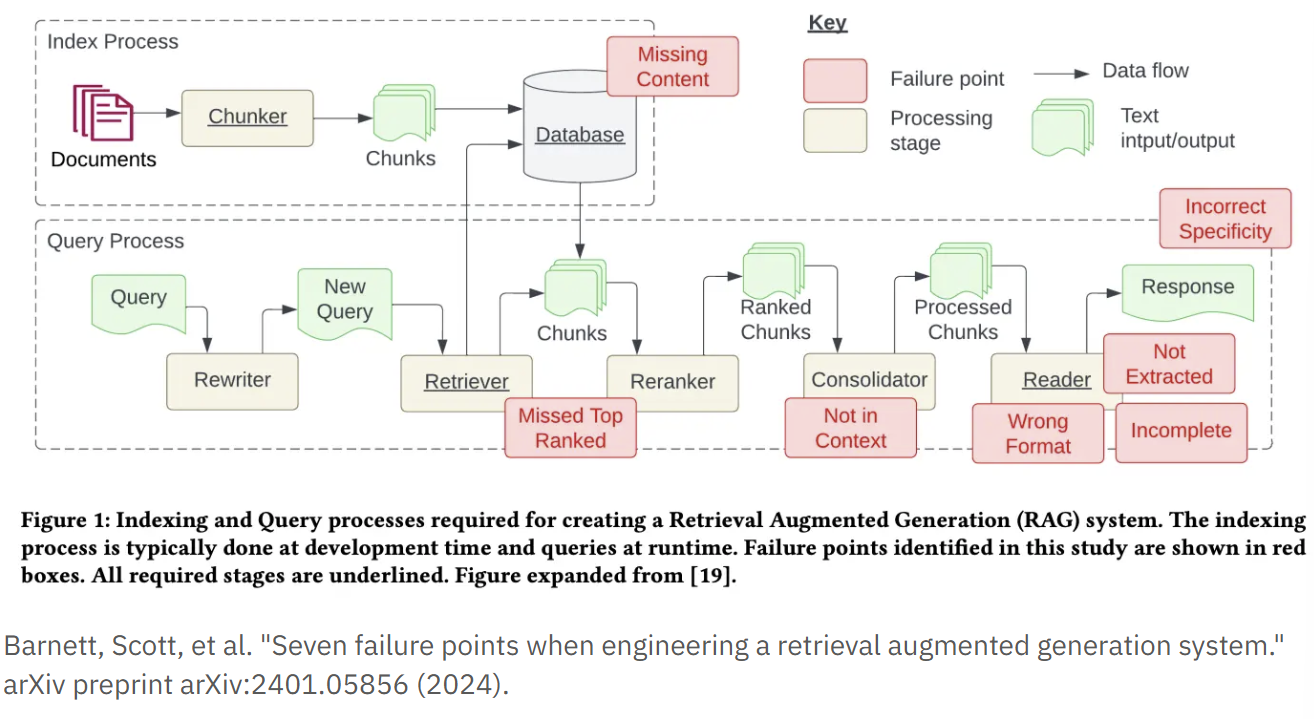
이외에도 retrieval 과정에서 vector DB에 저장된참고 자료를 제대로 가져오지 못한다던지, 사용자 입력에 대한 불완전한 응답을 제공한다던지 등 여러 한계가 존재한다. 이와 같은 기존 벡터 기반의 RAG 한계점을 보완하기 위해 지식 그래프 개념 기반의 GraphRAG가 등장했다.
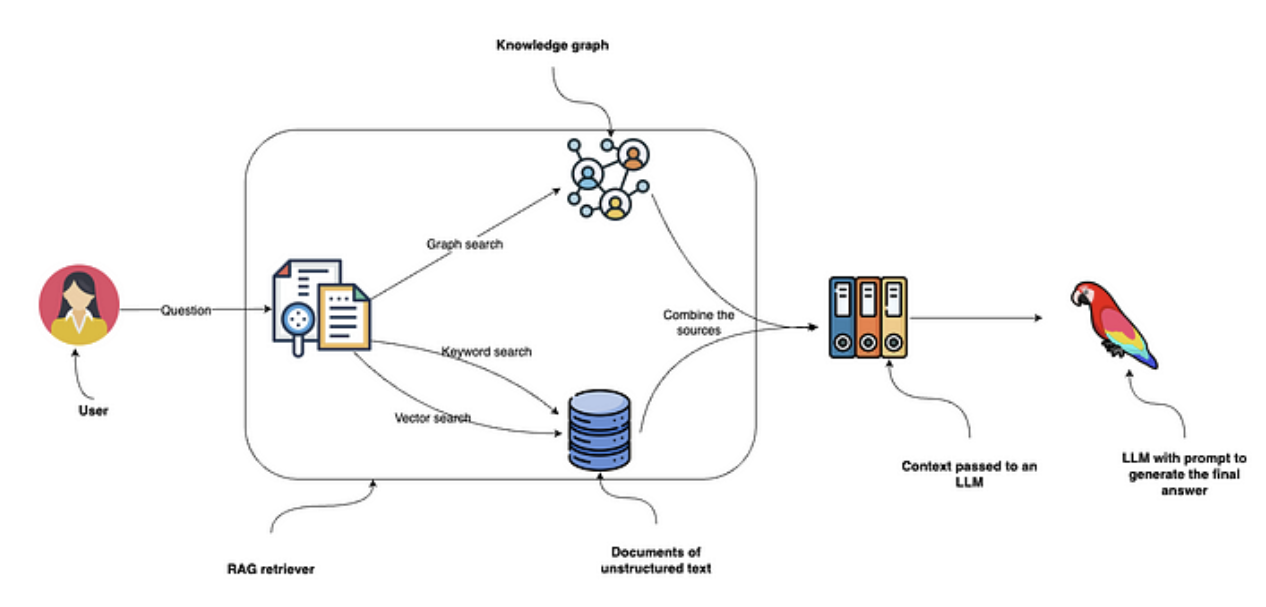
✅ Neo4j
지식 그래프(Knowledge Graph)를 구축해 지식을 검색하는 형태의 GraphRAG는 구조화된 데이터 엔티티(entity) 간 관계를 관리하고 활용하기 용이하다. 특히 그래프 형태로 상호 연결된 정보(노드, 엣지 등)들을
표현하고 저장하는데 지식 그래프의 데이터 구조와 GraphRAG 방식은 굉장히 효율적으로 동작할 수 있다. 그리고 이번엔 이 그래프 구조를 잘 표현하고 저장할 수 있는 Neo4j와의 결합을 해보고자 한다.
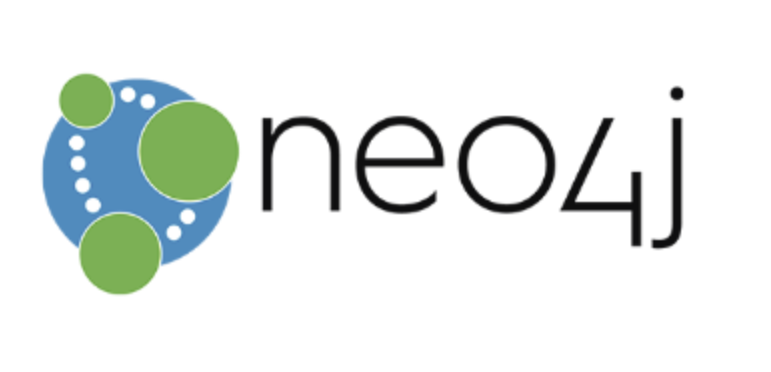
특징
1. 대규모 그래프 분석 및 데이터 시각화를 지원하는 확장성이 뛰어난 그래프 데이터베이스
2. Cypher 쿼리 언어, 내장된 시각화 도구 및 커뮤니티 지원
2️⃣ LangGraph
지난 포스트 중, langchain 에 대해 간단한 소개를 한 적이 있다. https://jhklee-coder.tistory.com/88
LangChain (랭체인)
랭체인(LangChain)은 대규모 언어 모델과 앱의 통합을 간소화하는 SDK*다. 최근 LLM 모델의 부상과 함께 그 활용성의 측면에서 랭체인의 범용성 또한 퍼지고 있다. 새로운 오픈소스 모델의 출시나 상
jhklee-coder.tistory.com
최근 기존의 LLM 통합과 체인을 구성하는 LangChain 기본 기능을 넘어 더 복잡한 내부 워크플로우와 정교한 의사결정 프로세스를 요구하는 경우가 많아지고 있다. 기존의 간단한 체인 구조와 애플리케이션을 구성하는데 중점을 두었던 Langchain의 한계를 좀 더 보완하고, 더 복잡한 프레임워크를 제공하는 것이 바로 LangGraph다. (이번 인턴 활동에서 가장 많이 활용해야 하는 영역이 바로 graph이기 때문에, LangGraph 라이브러리의 활용은 필수가 될 듯하다...)
LangGraph vs LangChain
주요 목적 | 복잡한 워크플로우 및 의사결정 프로세스 구현 | LLM 통합 및 체인 구성 |
구조 | 그래프 기반 | 체인 및 에이전트 기반 |
상태 관리 | 명시적이고 세밀한 제어 | 암시적이고 자동화된 관리 |
유연성 | 높음 (커스텀 로직 쉽게 구현) | 중간 (미리 정의된 컴포넌트 중심) |
학습 곡선 | 상대적으로 가파름 | 상대적으로 완만함 |
용도 | 복잡한 AI 시스템, 다중 에이전트 | 간단한 LLM 애플리케이션, RAG |
출처 - https://wikidocs.net/261585
✅ LangGraph의 주요 특징
1. 그래프 구조의 WorkFlow
2. 명시적인 상태 관리 (State Management)
3. 다중 에이전트 시스템 (Multi-agent)
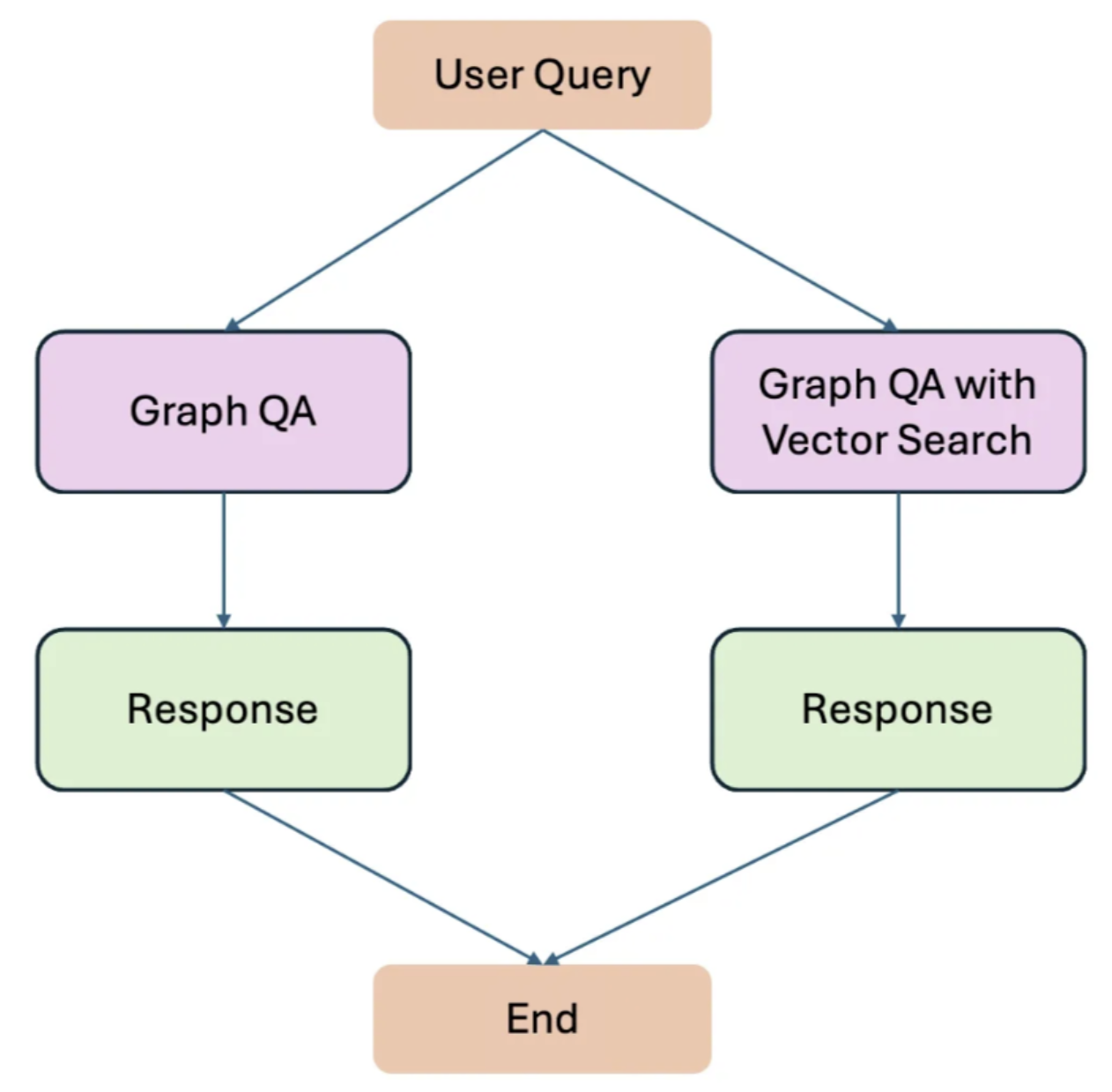
3️⃣ GraphRAG + LangGraph + Neo4j
✅ Neo4j 인스턴스 생성 및 Data Ingestion
1. langchain 및 neo4j 라이브러리 설치
# 라이브러리 및 패키지 설치
!pip install langchain
!pip install langchain_openai
!pip install neo4j
!pip install pyvis
2. Neo4j 인스턴스 생성
# Neo4j Sandbox Web browser DB 사용 예정
from langchain_community.graphs import Neo4jGraph
graph = Neo4jGraph(url = "bolt://...", username = "neo4j", password = "...", refresh_schema = False)
3. data를 그래프 구조로 ingest 하기 위한 함수 정의
import csv
import numpy as np
from neo4j import GraphDatabase
# Node : Retailer, Product, Supplier, Distributor, Manufacturer
NODES_CSV = "C:/LangGraph_Tutorial/.../nodes.csv"
# Edge : supplies, manufactures, offers
RELATIONSHIPS_CSV = "C:/LangGraph_Tutorial/.../relationships.csv"
# 데이터를 ingestion 하기 위한 함수
def get_label_for_type(node_type):
mapping = {
"Supplier": "Supplier", # 공급자
"Manufacturer" : "Manufacturer", # 생산자
"Distributor" : "Distributor", # 유통업자
"Retailer" : "Retailer", # 소매업자
"Product" : "Product" # 제품
}
return mapping.get(node_type, "Entity")
# 노드 정보 적재
def ingest_nodes(driver):
with driver.session() as session:
with open(NODES_CSV, mode = 'r', encoding = "utf-8") as f:
reader = csv.DictReader(f)
for row in reader:
node_id = row['id:ID']
name = row['name']
node_type = row['type']
location = row['location']
supply_capacity = np.random.randint(1000, 50001)
description = row['description']
label = get_label_for_type(node_type)
if location.strip():
# cypher 쿼리
query = f"""
MERGE (n:{label} {{id:$id}})
SET n.name = $name, n.location = $location,
n.description = $description, n.supply_capacity = $supply_capacity
"""
params = {
"id": node_id,
"name" : name,
"location" : location,
"description" : description,
"supply_capacity" : supply_capacity
}
else :
query = f"""
MERGE (n:{label} {{id:$id}})
SET n.name = $name
"""
params = {"id": node_id, "name": name}
session.run(query, params)
# 엣지(관계) 정보 적재
def ingest_relationships(driver):
with driver.session() as session:
with open(RELATIONSHIPS_CSV, mode='r', encoding='utf-8') as f:
reader = csv.DictReader(f)
for row in reader:
start_id = row[':START_ID']
end_id = row[':END_ID']
rel_type = row[':TYPE']
product = row['product']
if product.strip():
# cypher 쿼리
query = f"""
MATCH (start {{id:$start_id}})
MATCH (end {{id:$end_id}})
MERGE (start)-[r:{rel_type} {{product:$product}}]->(end)
"""
params = {
"start_id": start_id,
"end_id": end_id,
"product": product
}
else:
query = f"""
MATCH (start {{id:$start_id}})
MATCH (end {{id:$end_id}})
MERGE (start)-[r:{rel_type}]->(end)
"""
params = {
"start_id": start_id,
"end_id": end_id
}
session.run(query, params)
def create_indexes(driver):
with driver.session() as session:
for label in ["Supplier", "Manufacturer", "Distributor", "Retailer", "Product"]:
session.run(f"CREATE CONSTRAINT IF NOT EXISTS FOR (n:{label}) REQUIRE n.id IS UNIQUE")
4. Neo4j Sandbox web browser DB 연결 후 ingestion 수행
driver = GraphDatabase.driver(uri = "bolt://...", auth = ("neo4j", "..."))
create_indexes(driver)
ingest_nodes(driver)
ingest_relationships(driver)
print("Data ingestion complete!")
driver.close()
# => Data ingestion complete!

✅ Langchain 프레임워크 결합
1. Vector Embeddings 결합
import os
os.environ["OPENAI_API_KEY"] = "sk-..." # openai api key 입력
2. 구조화된 데이터 검색과 구조화되지 않은 텍스트 검색을 결합
from langchain_openai import OpenAIEmbeddings
from langchain_community.vectorstores import Neo4jVector
# neo4j 에 embedding 된 schema 구조.
embedding = OpenAIEmbeddings(model="text-embedding-3-small")
neo4j_vector = Neo4jVector.from_existing_graph(
url = "bolt://...",
username = "neo4j",
password = "...",
embedding=embedding,
index_name="supply_chain",
node_label="Supplier", # embedding 저장소를 supplier 노드에 제한
text_node_properties=["description"],
embedding_node_property="embedding",
)
=> Neo4j에서 semantic similarity search를 통해 그래프 형태의 구조화된 데이터를 검색 + 구조화되지 않은 텍스트 데이터(벡터)의 검색 기능을 결합.
✅ 구조화된 Query 처리를 위한 Tooling 작업
1. 숫자 필터링, 공급업체 계산, 목록 작성 등을 위한 도구 정의
# 입력 스키마 정의
from pydantic import BaseModel, Field
from typing import Optional, Dict, List
# 공급업체의 카운팅을 위한 class이자 Tool 정의
class SupplierCountInput(BaseModel):
min_supply_amount: Optional[int] = Field(
description="Minimum supply amount of the suppliers" # Supplier의 최소 공급량
)
max_supply_amount: Optional[int] = Field(
description="Maximum supply amount of the suppliers" # Supplier의 최대 공급량
)
grouping_key: Optional[str] = Field(
description="The key to group by the aggregation", # 최종 집계를 위한 키
enum=["supply_capacity", "location"]
)
2. 공급업체 계산을 위한 함수 정의
import re
from langchain_core.tools import tool
def extract_param_name(filter: str) -> str:
pattern = r'\$\w+'
match = re.search(pattern, filter) # filter 문자열에서 패턴을 검색
if match:
return match.group()[1:]
return None
@tool("supplier-count", args_schema=SupplierCountInput) # -> 도구 이름을 supplier-count tool로 등록
def supplier_count(
min_supply_amount: Optional[int], # 최소 공급량 필터
max_supply_amount: Optional[int], # 최대 공급량 필터
grouping_key: Optional[str],
) -> List[Dict]: # => return 값 : 공급업체 집계 리스트
"""Calculate the count of Suppliers based on particular filters"""
# -> 필터 조건 정의
filters = [
("t.supply_capacity >= $min_supply_amount", min_supply_amount), # 최소 공급량 조건
("t.supply_capacity <= $max_supply_amount", max_supply_amount) # 최대 공급량 조건
]
# condition에 포함된 변수명, 값을 매핑
params = {
extract_param_name(condition): value
for condition, value in filters if value is not None
}
# None이 아닌 조건들을 AND로 연결, Where 절 생성
where_clause = " AND ".join(
[condition for condition, value in filters if value is not None]
)
# 기본 cypher 쿼리 생성
cypher_statement = "MATCH (t:Supplier) " # "Supplier" 노드 매칭
if where_clause:
cypher_statement += f"WHERE {where_clause} "
return_clause = (
f"t.{grouping_key}, count(t) AS supplier_count"
if grouping_key
else "count(t) AS supplier_count"
)
cypher_statement += f"RETURN {return_clause}"
print(cypher_statement) # Debugging output
return graph.query(cypher_statement, params=params)
1) extract_param_name 함수
- 필터 조건에서 매개변수 이름을 추출.
- $min_supply_amount와 같은 조건에서 변수 이름을 가져옴.
2) supplier_count 함수
- 주어진 필터를 기반으로 공급업체를 세는 Cypher 쿼리를 생성.
- 위치와 같은 속성으로 결과를 그룹화(선택 사항).
3. 공급업체 목록 도구 정의
class SupplierListInput(BaseModel):
sort_by: str = Field(
description="How to sort Suppliers by supply capacity", enum=['supply_capacity']
)
k: Optional[int] = Field(
description="Number of Suppliers to return"
)
description: Optional[str] = Field(
description="Description of the Suppliers"
)
min_supply_amount: Optional[int] = Field(
description="Minimum supply amount of the suppliers"
)
max_supply_amount: Optional[int] = Field(
description="Maximum supply amount of the suppliers"
)
4. 공급업체 데이터를 검색하고 정렬하는 도구 정의
@tool("supplier-list", args_schema=SupplierListInput) # supplier-list 라는 tool로 등록.
def supplier_list(
sort_by: str = "supply_capacity", # 공급 능력에 따라 정렬
k : int = 4,
description: Optional[str] = None,
min_supply_amount: Optional[int] = None,
max_supply_amount: Optional[int] = None,
) -> List[Dict]:
"""List suppliers based on particular filters"""
# Handle vector-only search when no prefiltering is applied
# -> description이 주어지고, 다른 필터링 조건이 없을 시 벡터 유사성 검색을 수행.
if description and not min_supply_amount and not max_supply_amount:
return neo4j_vector.similarity_search(description, k=k)
filters = [
("t.supply_capacity >= $min_supply_amount", min_supply_amount),
("t.supply_capacity <= $max_supply_amount", max_supply_amount)
]
params = {
key.split("$")[1]: value for key, value in filters if value is not None
}
where_clause = " AND ".join([condition for condition, value in filters if value is not None])
cypher_statement = "MATCH (t:Supplier) "
if where_clause:
cypher_statement += f"WHERE {where_clause} "
# Sorting and returning
cypher_statement += " RETURN t.name AS name, t.location AS location, t.description as description, t.supply_capacity AS supply_capacity ORDER BY "
if description:
cypher_statement += (
"vector.similarity.cosine(t.embedding, $embedding) DESC " # 코사인 유사도를 기반으로 정렬
)
params["embedding"] = embedding.embed_query(description)
elif sort_by == "supply_capacity":
cypher_statement += "t.supply_capacity DESC "
else:
# Fallback or other possible sorting
cypher_statement += "t.year DESC "
cypher_statement += " LIMIT toInteger($limit)"
params["limit"] = k or 4
print(cypher_statement) # Debugging output
data = graph.query(cypher_statement, params=params)
return data
- 공급업체 나열
- 공급 능력별 정렬
- 능력별 필터링
- description 제공 시 벡터 검색을 수행하는 도구
✅ LangChain & LangGraph 과의 결합
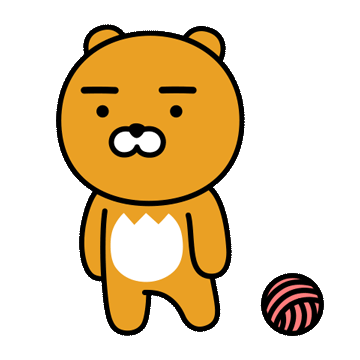
이번 주는 RAG 에 대한 주요 개념과 Graph를 활용한 RAG 기법의 핵심 기능 확장, 나아가 LangGraph를 활용한 파이프라인 구현을 해보았다. 아직 업무에 대한 이해와 LinkBrain 프로젝트의 방향성을 스스로 확립하지 못했다는 생각이 든다. 아직은 열심히 프레임워크와 툴, 핵심 로직 구현을 위한 공부에 열중할 때..!!!!
인턴 일기는 다음 글에 이어집니다...
3️⃣ Reference
https://medium.com/globant/langgraph-ai-agents-with-neo4j-knowledge-graph-7e688888f547
LangGraph AI Agents with Neo4j Knowledge Graph
A RAG System Built for Both Semantic Search and Structured Data Queries
medium.com
https://python.langchain.com/docs/tutorials/graph/
Build a Question Answering application over a Graph Database | 🦜️🔗 LangChain
In this guide we'll go over the basic ways to create a Q&A chain over a graph database. These systems will allow us to ask a question about the data in a graph database and get back a natural language answer. First, we will show a simple out-of-the-box opt
python.langchain.com
https://langchain-ai.github.io/langgraph/
Home
🦜🕸️LangGraph ⚡ Building language agents as graphs ⚡ Overview LangGraph is a library for building stateful, multi-actor applications with LLMs, used to create agent and multi-agent workflows. Check out an introductory tutorial here. LangGraph is
langchain-ai.github.io
https://github.com/sgautam666/my_graph_blogs/tree/main/neo4j_rag_with_langGraph
my_graph_blogs/neo4j_rag_with_langGraph at main · sgautam666/my_graph_blogs
A collection project that uses graph technology. Contribute to sgautam666/my_graph_blogs development by creating an account on GitHub.
github.com
'Experience' 카테고리의 다른 글
스타트업 인턴 일기 [04화] (0) | 2025.02.14 |
---|---|
스타트업 인턴 일기 [03화] (1) | 2025.02.06 |
스타트업 인턴 일기 [01화] (0) | 2025.01.15 |
경희대학교 데이터 분석 & AI 동아리 KHUDA 4th [교내활동] (1) | 2023.12.26 |
OUTTA 부트캠프(최종 프로젝트) / 후기 및 활동 내역 [대외활동] (0) | 2023.12.23 |